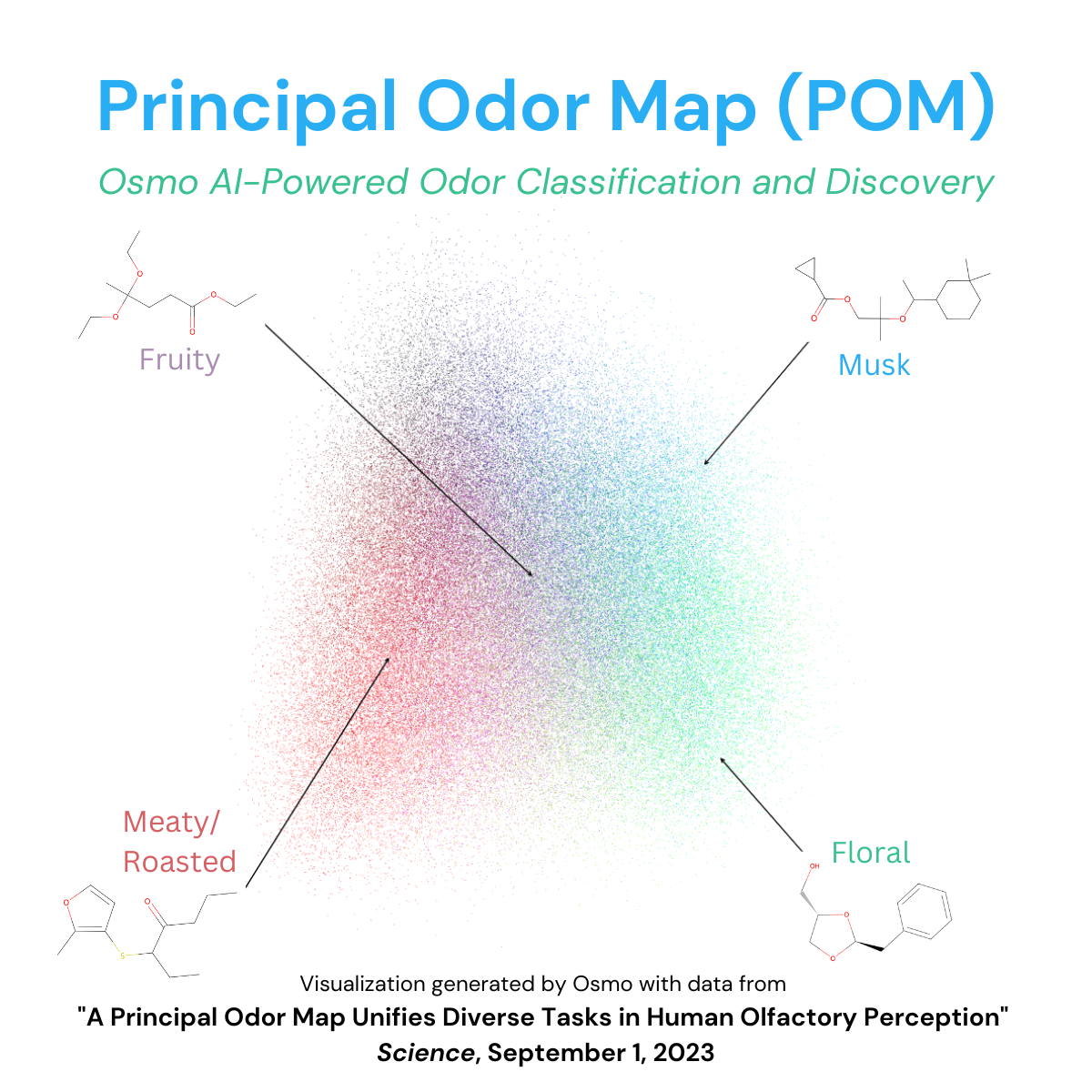
Science Paper Shows Osmo AI Passes the Sniff Test
At Osmo, we’re on a mission to digitize scent to improve human health and happiness. If we can do this, we imagine a world where people live longer, healthier, happier lives. We hope the technologies we build will also unleash new kinds of experiences and creativity.
In an important step towards digitizing scent, today our foundational research paper “A Principal Odor Map Unifies Diverse Tasks in Human Olfactory Perception” published in Science. Rick Gerkin, Osmo’s head of neuroscience, and I co-authored the paper with researchers from the Monell Chemical Senses Center, the University of Reading, and Arizona State University.
In short, the paper validates the idea that it’s now possible to apply machine learning to quantify, digitize, and engineer scent. A core problem preventing us from digitizing scent is something that the other senses already have – a map. RGB is a three-dimensional map of all colors, and low- to high-frequency is a one-dimensional map that lets you describe any sound and transmit any song. Scent has never had a map. While there are three channels of color information in your eye, there are over 300 channels of odor information in your nose. That’s the power and the complexity of the olfactory system; it’s designed to detect an enormous diversity of molecules. We view our discovery of the Principal Odor Map (POM) as the olfactory version of RGB. Our paper proves the usefulness of the map we built by predicting the scent of molecules that had never before been smelled.
Because of the complexity of scent, we had to use novel datasets and AI to discover the map, and found that it has the precision and predictability to pass what I like to call an “Odor Turing Test”: the model performed better than the average human panelist on 53% of the molecules tested, predicting odor solely from molecular structure.
On a personal note, I’ve been obsessed with the question of why the world smells the way it does for most of my life. I started studying it professionally after I watched a TED talk given by Luca Turin in 2008, when he highlighted that scientists don’t know how to look at a molecule and predict what it will smell like. We know why an apple looks red, we know why the crash of a cymbal is bright and loud, but why don’t we know why a molecule smells of cut grass, or cinnamon, or nothing at all? I was in college studying neuroscience at the time, and I couldn’t quite fathom how something that seems so simple would still be so mysterious to all of neuroscience. I’ve spent the next 15 years trying to answer this question, and this paper is a major milestone in this quest.
Can a computer predict smell based on molecular structure?
More specifically, we wanted to find out if a computer could predict smells based on molecular structure as well as or better than a human could detect them.
To do this we:
- Built a machine-learning model using graph neural networks (GNNs). To map how molecular structure corresponded with the way molecules smell, we trained the model using a dataset of 5,000 known compounds that were paired with the smell labels they evoked, such as "fruity," "floral," “cheesy,” or "minty."
- Froze the model in place and then selected and tested it with 400 compounds. These compounds were selected to be a) structurally different from each other, b) structurally different from anything in our dataset or used in the fragrance industry, and c) predicted to smell different from each other. The idea was to see how the model predicted the scents of truly new molecules compared to 15 human panelists.
- Put the model through a more stringent “adversarial” test by identifying sets of molecules where structural similarity and empirical perceptual similarity (i.e., smell) are mismatched. This is adversarial because such models use structure as the input, so any discordance between structure and perception should make it hard for models to get the perceptual prediction right. Facing this maximally difficult challenge, our model made perceptual predictions that matched the empirical data 50% of the time, vs <20% of the time for the baseline model.
- Examined the Principal Odor Map’s generalizability more broadly by asking whether it could assess olfactory properties that weren’t used to train Osmo’s model – such as odor strength and perceptual similarity – for 500,000 potential scent molecules.
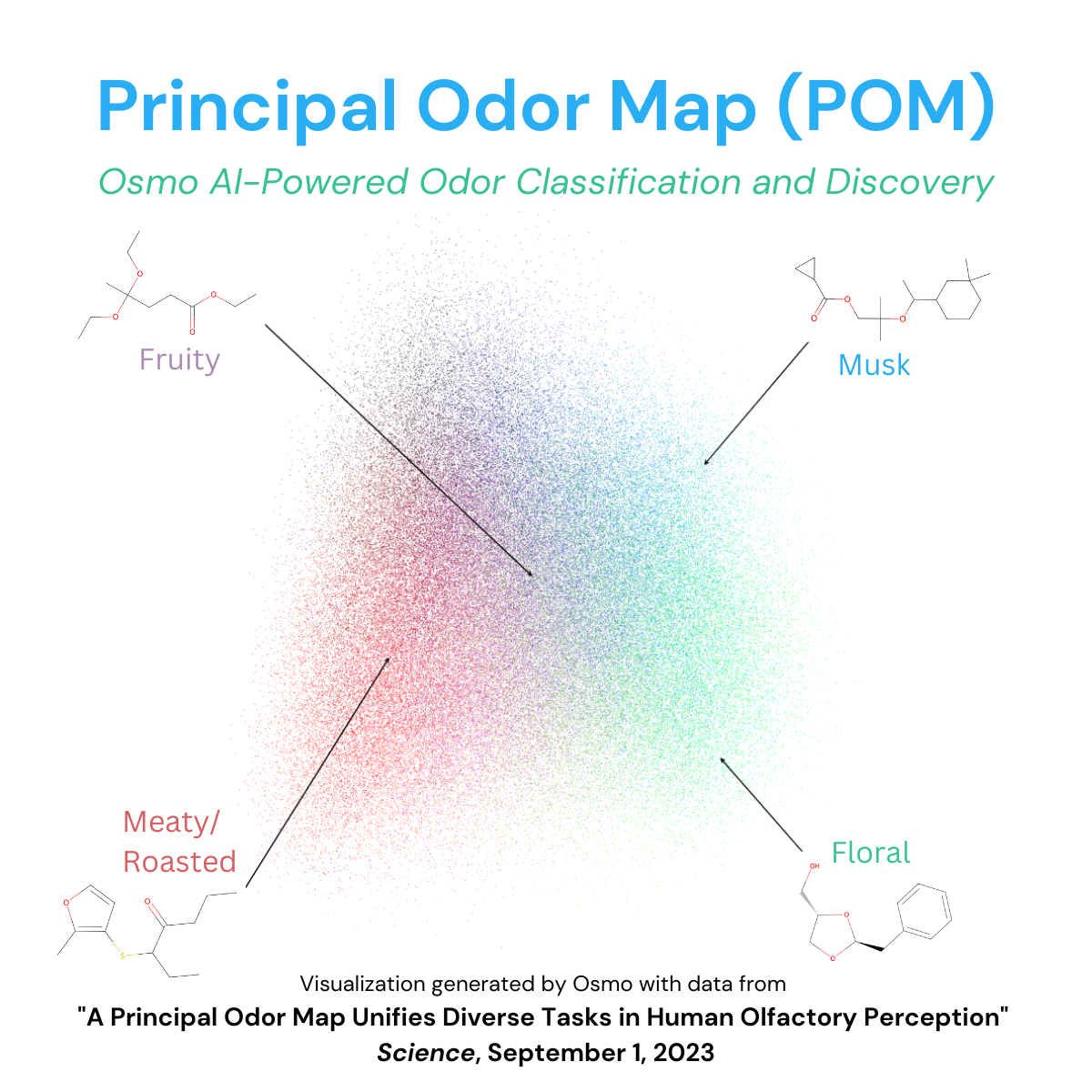
Key findings: a higher order odor map
This was the first use of graph neural networks for olfactory prediction, validated on novel molecules and tested by a trained panelist’s sense of smell. It showed that the team was able to generate a map of odor that works, and captures the higher-order structure of scent.
- Working with 15 human panelists from an ethnically diverse population and using data from previous work, we could account for similarities and differences in human perception. Using data from previous work, we saw both cross-cultural differences and discovered that smell shared many commonalities across different backgrounds.
- We also trained panelists. Each panelist received an odor reference kit with at least one reference odor for each term in the lexicon. They kept the reference kit throughout the project and could refer back to it at any time. We were not relying on them having the same interpretation of the word – we were teaching them which smell corresponds to that word.
- Comparing the model’s performance to that of individual participants, who rated scents using a lexicon of odor descriptors, it achieved better predictions of the consensus – the average of the group’s odor ratings – than any single participant in the study for over half of the molecules tested. It achieved this feat by making predictions only using molecular structure, reaching performance just beyond that of the average trained panelist, in our Odor Turing Test. That means, if you were to add a new person to a panel or choose the predictions from our odor map, you’d choose our odor map.
- We compared our Principal Odor Map (POM) to a pre-existing baseline, called “Morgan fingerprints.” The POM achieved super-human performance, whereas Morgan fingerprints did not.
- The model’s predictions aligned with panelist responses, correctly identifying that many apparently structurally dissimilar molecules have counter-intuitively similar smells. It performed better than a baseline chemoinformatic model across multiple datasets, which showed that our POM works in multiple areas of olfaction, not just to address the problem at hand.
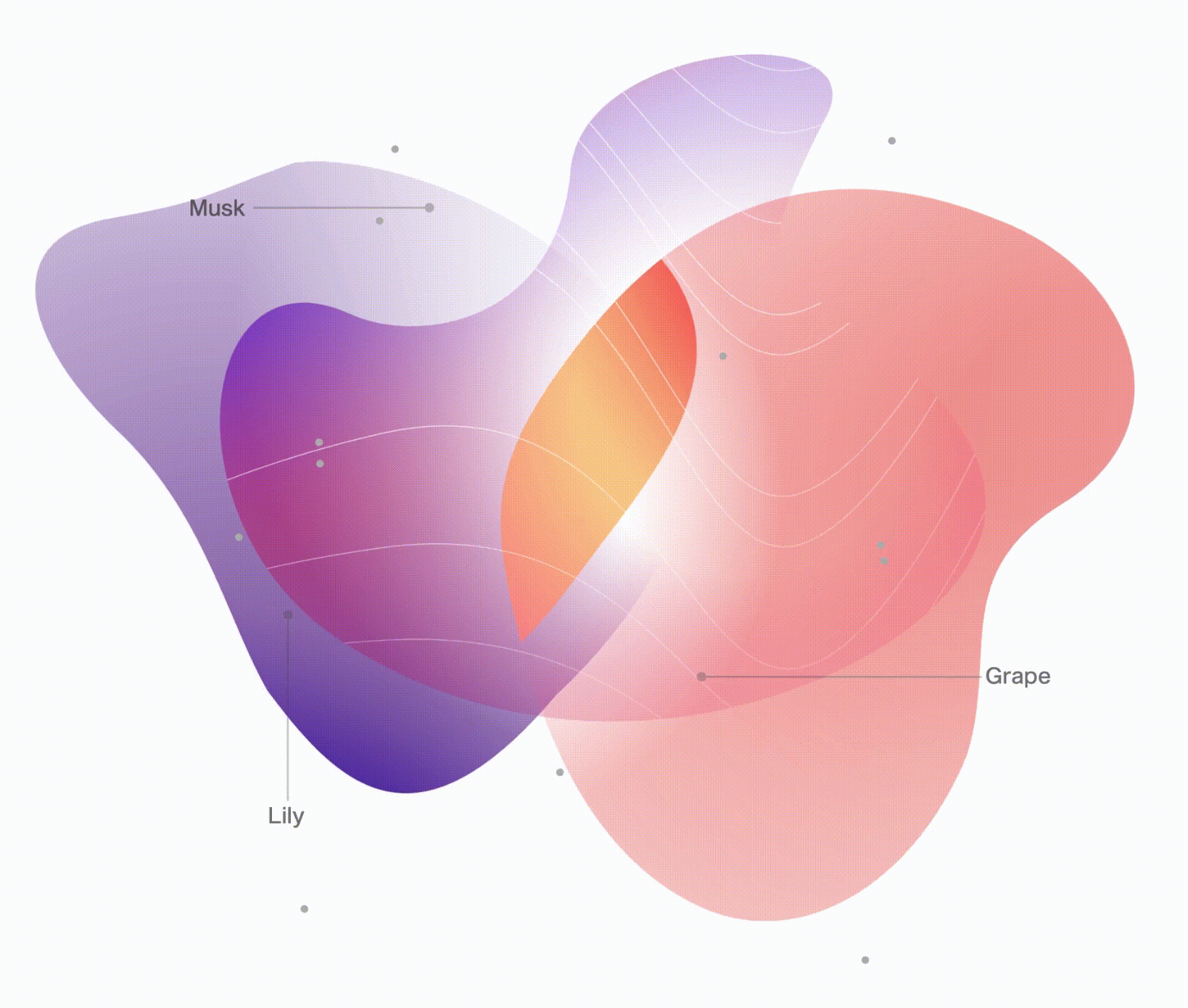
What this means for Osmo
Our model performs over 3x better than the standard scent ingredient discovery process used by major fragrance houses, and is fully automated. This discovery is the foundation of everything that Osmo will do next. Moving forward, we’ll be using this technology to discover the next generation of safe but powerful fragrance ingredients both in our own laboratories and in partnership with leading industry players. It’s our goal in the work ahead of us to build a strong scientific and commercial foundation for our mission of digitizing smell to improve human health and happiness. If we can make the fragrances we use every day safer and more potent (so we use less of them), we’ll help the health of everyone, and also the environment.
But, there are still many mysteries that remain. First, we don’t predict how intensely a molecule smells, just what it smells like. Can we predict which molecules will be strong, and which will be weak? Second, we only make predictions on single molecules, but you always smell mixtures of molecules in real life. Can we predict how molecules will smell when blended together? Third, we’re making predictions on molecular structures that have already been digitized, but in life you directly experience real physical material, not bits of information. Can we predict smell directly from chemical sensor measurements? Fourth, if we can achieve all of the previous abilities, can we digitize a scent in one place and time, and then faithfully replicate it in another?
There is much work to be done to give computers a sense of smell, and many potential applications of digital olfaction.
The future is safer products
In some ways, we see AI in drug discovery as a precedent for ways that AI will revolutionize olfaction. Similar to how the advent of AI is changing the way drug hunters find new candidates that are more likely to succeed in the clinic, we see AI augmenting the role of synthetic chemists and master perfumers, the scent hunters who comb through myriad molecules to create the scents that are an integral part of our lives.
Ultimately, we want to create new and safer products. A biodegradable molecule will break into pieces that can be recycled into nature. A problematic molecule will stay in its current form or won't break down, or it will break down into something harmful. So we want to be very careful when we design these molecules.
Our map of odor is the foundation for our broader ambitions. There are signals in the scents from our bodies and in the world around us that capture memories, emotions, and signals about our health and well-being. If we can build on our insights to develop systems capable of replicating what our nose, or what a dog’s nose can do (smell diseases!), we can spot disease early, prevent food waste, capture powerful memories, and more. If computers could do these kinds of things, people would live longer lives – full stop. Digitizing scent could catalyze the transformation of scent from something people see as ephemeral to enduring.