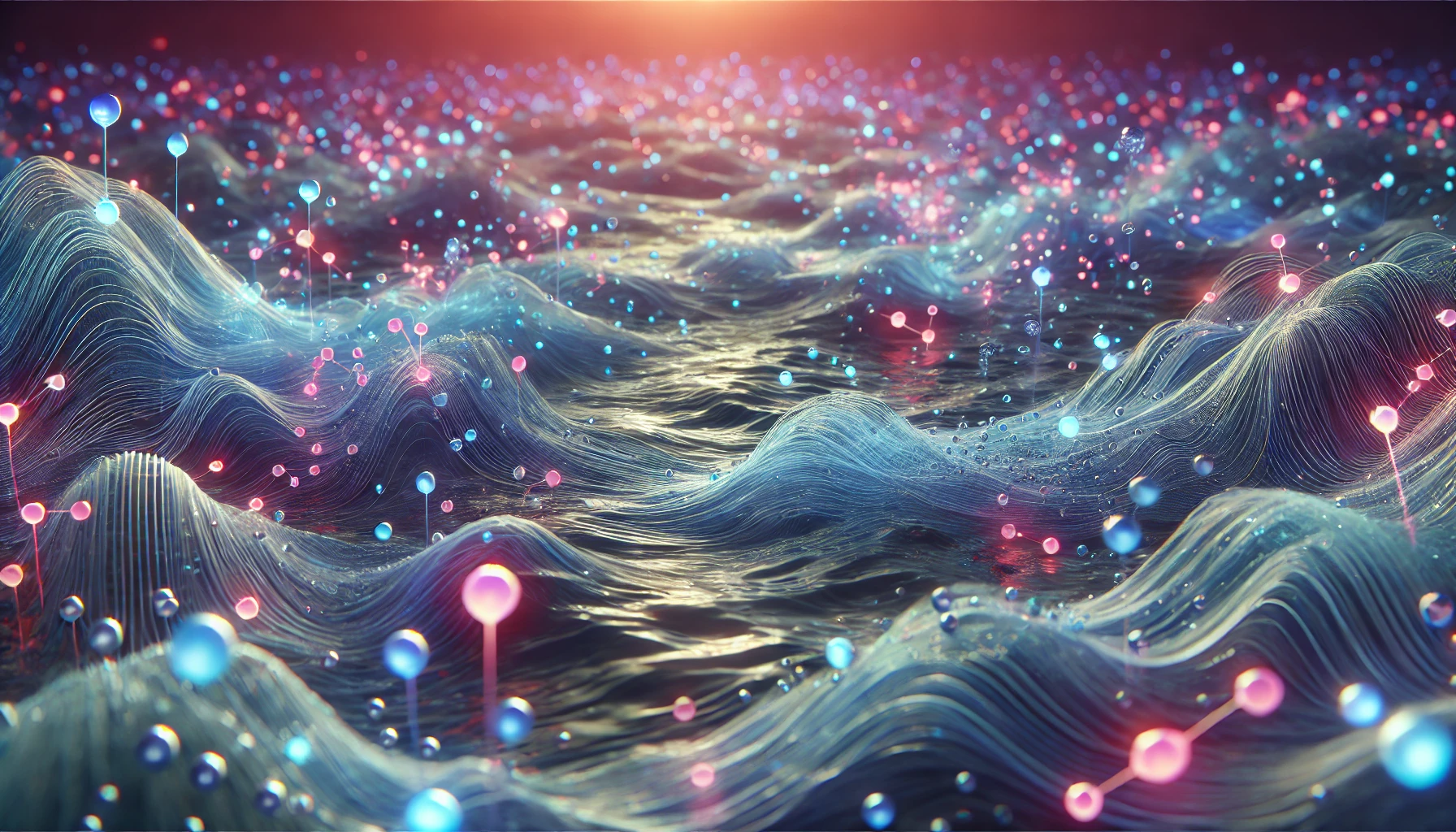
[Tech Blog] Revolutionizing Scent Creation
How Osmo finds new smells in an ocean of a billion molecules.
Intro

Machine learning has revolutionized drug discovery by enabling rapid and efficient exploration of vast virtual chemical libraries. By employing ML algorithms, researchers can quickly identify potential drug candidates and optimize their properties based on various screening assays. This technology significantly accelerates the traditional drug development process by reducing the time needed for synthesis and testing, ultimately bringing promising drug compounds to market more efficiently.
Osmo wants to bring this same innovation to a very different industry: Fragrances. The major limitation to this approach is that prior to the foundational research done when part of the Osmo team was at Google, there was no way to virtually predict the smell of compounds to allow for screening. With this new capability, Osmo can shift focus to screening all of chemical space, opening up vast amounts of possible new compounds that would be unknowable to the traditional fragrance houses.
The Platform
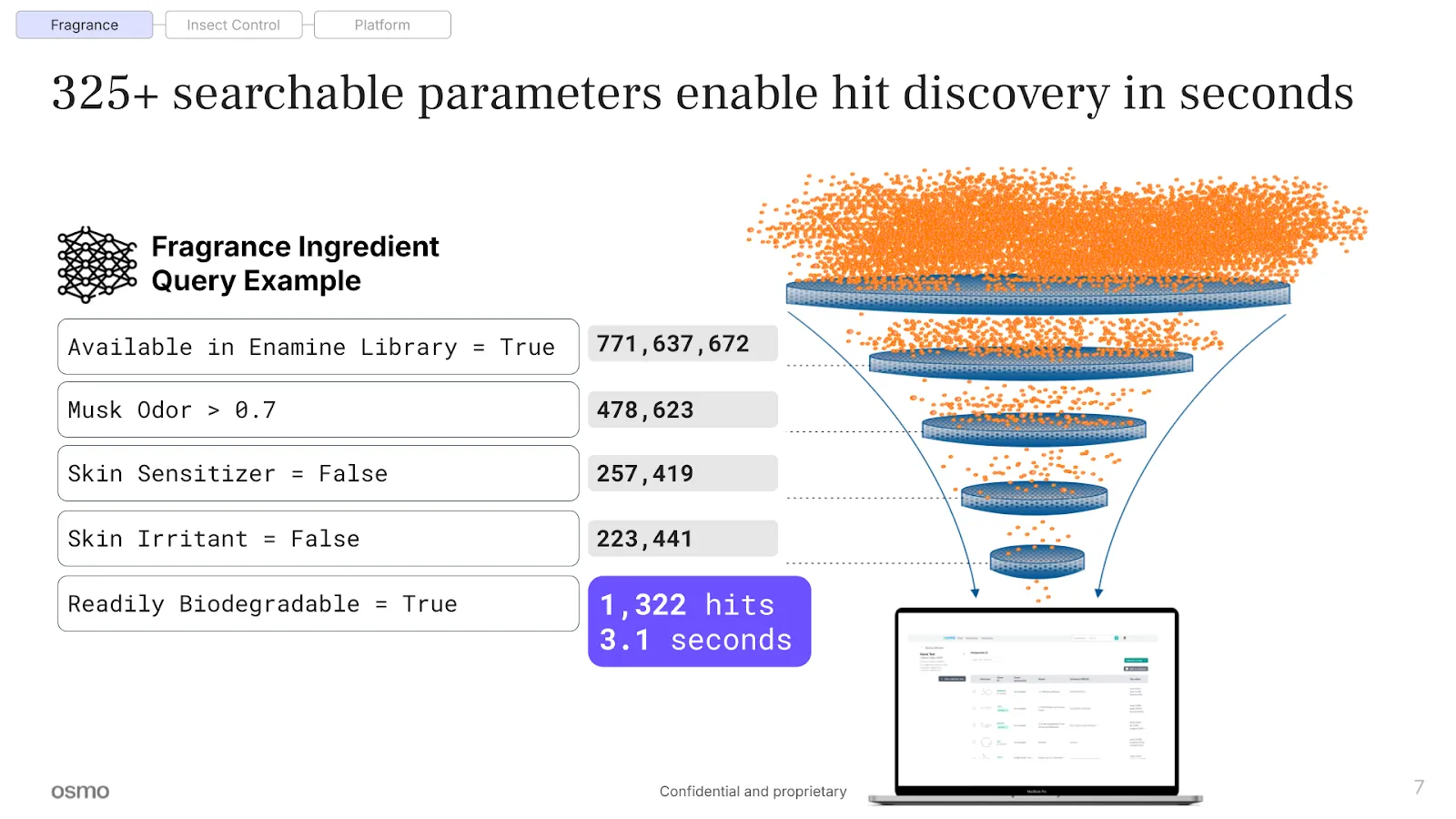
Osmo's utilizes Google Cloud Platform to streamline fragrance development. Osmo has constructed a virtual library of over a billion small molecules, specifically focusing on those suitable as building blocks for new scents. This massive library, combined with Osmo-specific canonicalization functions, ensures a unique and efficient exploration of potential fragrance molecules. All predictive data regarding odor characteristics, toxicity, and biodegradability are stored in BigQuery, allowing for rapid access and analysis, essential for keeping pace with the fast-moving consumer goods sector.
Osmo engineers created new extensions to BigQuery, allowing for greater ease to work with chemical data. The focus has been to bring many of the convenient functions you would find in a library like rdkit directly into BigQuery itself. This, combined with the serverless nature of BigQuery and Cloud Functions, allows a single query that involves complex molecule functional group filtering, distance metrics, and ranking to run in seconds over TBs of data.
From this point, Osmo leverages a mixture of internal and external tools to go from a virtual list of compounds to real vials in the lab that can be smelled and tested against the model. Like most of the Chemistry world, this involves occasionally Google Sheets to help the Scientists make comparisons between various choices. Like in the BigQuery example, Osmo Engineers have built custom plugins and extensions to Google Sheets that makes it much easier to work directly with Chemicals. This includes visualizations, chemical canonicalization, substructure search, and other model predictions.
The Future
At Osmo, we want to continue to push the boundaries of new smells and find new molecules that are both safer for the environment and for humans. We continue to make every step of this process faster, bigger, and easier for the Scientists to decide when a virtual molecule should be brought into the world for the first time. We believe that some of the tools we have built for Google Cloud Platform, in particular BigQuery and Google Sheets, are valuable to companies outside of Osmo. We hope to have more to say on that in the near future.